Hackers can access a patient's 3-D medical scans to add or remove images of malignant tumors, thus placing patients at risk of misdiagnoses. The new study, published by Ben-Gurion University of the Negev cybersecurity researchers, showed that the altered scans successfully deceived both radiologists and artificial intelligence algorithms used to aid diagnosis.
A 3-D CT (computerized tomography) scan combines a series of X-Ray images taken from different angles around the body and uses computer processing to create cross-sectional images (slices) of the bones, blood vessels and soft tissues. CT scan images provide more detailed information than standard X-Rays, and are used to diagnose cancer, heart disease, infectious diseases, and more. An MRI (magnetic resonance imaging) scan is similar, but uses powerful magnetic fields to diagnose bone, joint, ligament, and cartilage conditions. Deliberately tampering with the scans could aid insurance fraud, ransomware, cyberterrorism or even murder. Attackers can even automate the entire process in a malware which can infect the hospital's network.
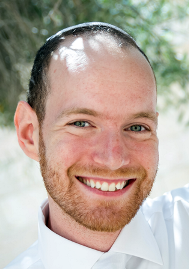
“Our research shows how an attacker can realistically add or remove medical conditions from CT and MRI scans," says Dr. Yisroel Mirsky (pictured above), lead researcher in BGU's Department of Software and Information Systems Engineering (SISE), project manager and cybersecurity researcher at BGU's National Cyber Security Research Center. “In particular, we show how easily an attacker can access a hospital's network, and then inject or remove (images of) lung cancer from a patient's CT scan."
The attacker has full control over the number, size and locations of the cancers while preserving the same anatomy from the original, full resolution 3-D image. This is a significant threat since 3-D medical scans are considered to provide more definitive evidence than preliminary 2-D X-Rays.
To demonstrate the feasibility of the attack, with permission, the researchers broke into the network of an actual hospital and intercepted every scan taken by a CT scanner.
“The scans were not encrypted because the internal network is usually not connected to the internet. However, determined intruders can still gain access via the hospital's Wi-Fi or physical access to the infrastructure," Dr. Mirsky says. “However, these networks are now being connected to the internet as well, which enables attackers to perform remote attacks."
To inject and remove medical conditions, the researchers used a deep learning neural network called a generative adversarial network (GAN). GANs have been used in the past to generate realistic imagery, such as portraits of non-existent people. The researchers showed how a 3-D conditional GAN can be used to efficiently manipulate high resolution 3-D medical imagery. The architecture (CT-GAN) uses two of these GANs: one trained to inject cancer and the other trained to remove cancer.
The BGU researchers verified the attack effectiveness by training CT-GAN to inject/remove lung cancer using free medical imagery off the internet. They hired three radiologists to diagnose a mix of 70 tampered and 30 authentic CT scans.
The radiologists misdiagnosed 99 percent of the altered scans showing malignant tumors, and 94 percent of altered images that had had cancerous images removed. After informing the radiologists of the attack, they still could not differentiate between the tampered and authentic images, misdiagnosing 60 percent of altered scans falsely showing tumors and 87 percent of those falsely showing no sign of tumor.
“In addition to the radiologists, we also showed how CT-GAN is an effective adversarial machine learning attack," Dr. Mirsky says. “Consequently, the state-of-the-art artificial intelligence lung cancer screening tools, used by some radiologists, are also vulnerable to this attack."
The researchers proposed some immediate countermeasures which can mitigate most of the threat. One solution is to enable encryption between the hosts in the hospital's radiology network. In addition, some hospitals can enable digital signatures so that their scanners sign each scan with a secure mark of authenticity. If this approach is followed, then administrators should ensure that proper signatures are being used and that the end devices are correctly verifying these signatures.
“Another method for testing the integrity of the images is to perform digital watermarking (DW), the process of adding a hidden signal into the image such that tampering corrupts the signal and thus indicates a loss of integrity," Dr. Mirsky says. “Unfortunately, the vast majority of medical devices and products currently do not implement DW techniques."
Other researchers that participated in the study include: Prof. Yuval Elovici, Ph.D., director of the Telekom Innovation Labs@BGU, director of Cyber@BGU; Tom Mahler, Ph.D. candidate and researcher in Cyber@BGU, and a member of the BGU SISE; and Prof. Ilan Shelef, M.D., Ph.D., director of the imaging department in Soroka University Medical Center and a member of BGU's Faculty of Health Sciences.
Media Coverage:
The Washington Post
The Jerusalem Post
PC Mag
Forbes
BBC