The paper "Stable Sample Compression Schemes: New Applications and an Optimal SVM Margin Bound" by Prof. Kontorovich from the Department of Computer Science at BGU and his co-author Dr. Steve Hanneke from Toyota Technological Institute at Chicago (TTIC), was selected as the winner of the best paper award at the prestigious International Conference on Algorithmic Learning Theory (ALT 2021).
In the paper the authors make use of the recently defined notion of a stable compression scheme, meaning that removing points from the training set which were not selected for the compression set does not alter the resulting classifier. They use this technique to derive a variety of novel or improved data-dependent generalization bounds for several learning algorithms. In particular, they proved a new margin bound for SVM, removing a log factor. The new bound is provably optimal. This resolves a long-standing open question about the PAC margin bounds achievable by SVM.
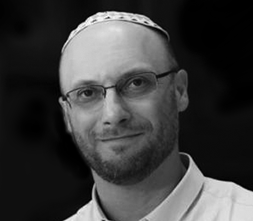