Speaker 01: Or Symonitz
Title: Predicting Dynamic Recovery Following Rehabilitation Treatment Using Graph-Theory Analysis of Resting State fMRI in Chronic Post-Stroke Subjects
Abstract:
Stroke is associated with damage to neural tissue and is the leading cause of long-term motor disability in adults. Dynamic balance impairments are one of the most debilitating outcomes of stroke, leading to increased falls and loss of mobility. The recovery of motor functions following stroke was shown to be affected by the initial brain impairment, passage of time, and dosage of rehabilitation treatment. We demonstrate here that a novel approach including machine learning and graph analysis applied on the resting state fMRI scans of stroke patients taken before a dynamic balance rehabilitation treatment can predict their recovery. Furthermore, we report that global features, describing the brain graph as a whole, are more informative than local features, describing individual regions, for predicting the success of a rehabilitation, reaching accuracy levels of 86% .
Furthermore, graph embedding using NetLSD Wave exhibits 86% and 80% accuracy when predicting the community balance and mobility (CBM) scale before and after the treatment respectively. These graph-theory based neural markers may contribute to enhancing recovery through improving the selection of rehabilitative treatments.
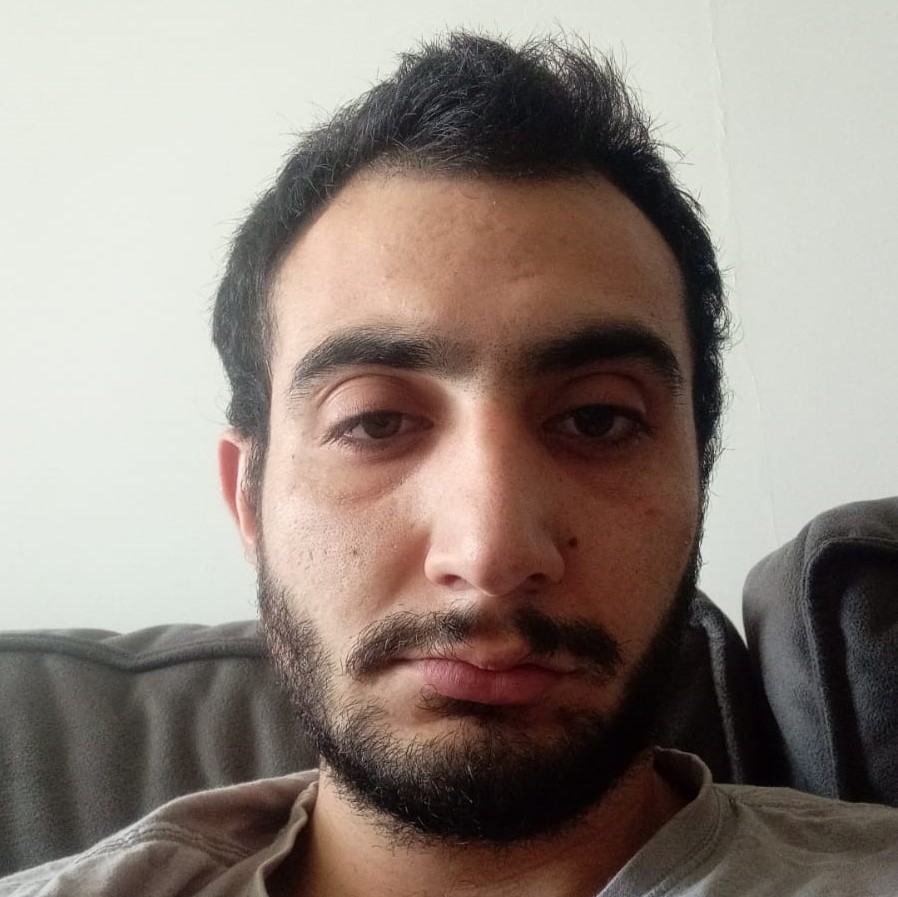
Bio:
Or Symonitz Is a Soldier and a master student in the department of Software and Information Systems Engineering, at Ben-Gurion University of the Negev (BGU), Beer-Sheva, Israel. Studying under the superposition of Dr. Rami Puzis and Dr. Lior Shmuelof from the Brain and Action Lab.
His research topics at prediction of balance rehabilitation of patients after a treatment among stroke patients.
Speaker 02: Shachar Wild
Title: Cost-Oriented Candidate Screening Using Machine Learning Algorithms
Abstract: Choosing the right candidates for any kind of position, whether it is for academic studies or for a professional job, is not an easy task, since each candidate has multiple traits, which may impact her or his success probability in a different way. Furthermore, admitting inappropriate candidates and leaving out the right ones may incur significant costs to the screening organization. Therefore, such a candidate selection process requires a lot of time and resources. In this paper, we treat this task as a cost optimization problem and use machine learning methods to predict the most cost-effective number of candidates to admit, given a ranked list of all candidates and a cost function. This is a general problem, which applies to various domains, such as: job candidate screening, student admission, document retrieval, and diagnostic testing. We conduct comprehensive experiments on two real-world case studies that demonstrate the effectiveness of the proposed method in finding the optimal number of admitted candidates.
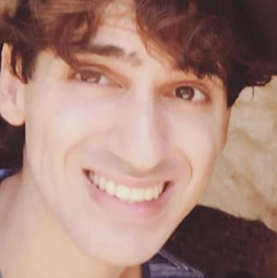
Bio:
Shachar Wild, M.Sc student in Software and Information Systems Engineering at BGU, specializing in Computational Learning & Big Data. B.Sc in Software and Information Systems Engineering was acquired at BGU. Currently working as a Data Scientist at SAP.