Thanks to a novel plant-growth model based on satellite and ground-based data, wheat farmers – particularly in dryland regions – have an improved new crop management tool enabling them to maximize field returns. The sophisticated model, which characterizes crop condition in real time, informs agriculturists whether application of nitrogen fertilizer is required, when best to harvest the crop, and whether irrigation might be economically viable in a dry year. Because satellite data covers vast regions, major governmental or agricultural organizations taking advantage of this model can provide hundreds of farmers with vital procedural approaches to maximize their incomes.
Prof. Arnon Karnieli and his team at the BIDR Remote Sensing Laboratory , collaborating with David J. Bonfil of the Agricultural Research Organization (ARO, Volcani Center), Israel Ministry of Agriculture and Rural Development, have developed the first crop growth model utilizing satellite data for aiding wheat farmers. Their initial work is most valuable for dryland farmers who rely solely on natural rainfall to irrigate their crops. Here, growers must know whether there was sufficient rain to produce high-quality grain. If not, farmers will prefer to cut down the stalks early in March before grain formation (or heading) and sell their crop as green forage. The new model gives farmers a simple yes/no answer to this question, information that would be much more difficult to obtain from field measurements.
The model involves determining the water and nitrogen (protein manufacturing) status in wheatflag leaves, the last mature leaf growing next to the grass inflorescence. These two factors are predictive of quantity and quality of the wheat grains eventually formed. Insufficient water during the grain filling stage may restrict grain yields and inhibit protein accumulation, while drought often inhibits starch accumulation, a condition preventing proper milling of flour. When nitrogen is low, formation of storage proteins (gliadin and glutenin) is lowered, yielding low-quality low-commercial-value grains. Under certain conditions, however, low flag-leaf nitrogen can be reversed by application of fertilizer, resulting in the production of acceptable-quality grain.
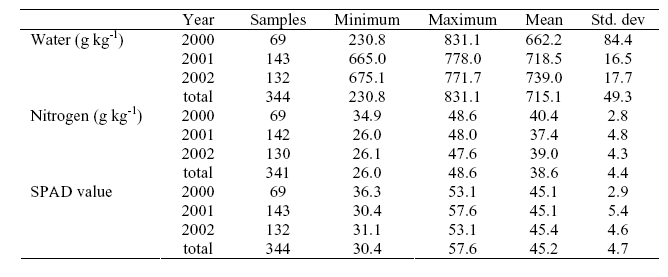 |
Water and nitrogen composition of wheat leaves used in NIR transmittance and reflectence studies, and their SPAD values. Each sample represents an average of 4 different flag leaves (4 cm long taken from the central leaf blade). For more information see Bonifil et al. 2005. |
A detailed study of the spectral properties of flag leaves from experimental grain fields and laboratory determinations of their water and nitrogen contents was carried out by the BIDR/ARO team. The researchers found that near infrared reflectance spectra from the leaves in the 1.100 – 2.498 micron wavelength region could be used for real-time analytical determination of flag-leaf water (FLW) and flag-leaf total nitrogen (FLN). By determining the FLW and FLN of plants just developing their inflorescence and obtaining meteorological forecasts of near-term precipitation levels, a Decision Support System, or DSS, built on the plant-growth model, evaluates this data and provides clear recommendations to farmers. Should the field be immediately harvested for hay? Should it be allowed to grow undisturbed to produce acceptable wheat? Or should nitrogen fertilizer be applied right before the next rain to ensure a profitable grain harvest?
In a large-scale experiment in 2000 and 2001, 344 experimental wheat plots in the semi-aridNegev received different schedules of fertilizer application and irrigation. In more than 80 percent of the plots, the proposed DSS correctly forecasted grain quality, quantity (test weight), and protein content. This novel remote-sensing approach can be applied to reducing the harvesting of shriveled, unmarketable grains, on one hand, and lead to improved grain protein, on the other. The agricultural authorities therefore have a new tool for advancing and expanding wheat farming in semi-arid regions.
DSS recommendations based on FLW and FLN at heading and grain quality parameters of rained (dry) and irrigated (Irr) wheat. DSS algorithm based on data from only two seasons 2000 and 2001. Groups marked with close symbols (+N) received N application after heading, regardless of the DSS recommendation. Broken lines represent premium and penalty baselines.
|